Supporting teachers to integrate AI in K–12 CS education
Teaching about artificial intelligence (AI) is a growing challenge for educators around the world. In our current seminar series, we are gaining insights from international computing education researchers on how to teach about AI and data science in the classroom. In our second seminar, Franz Jetzinger from the Technical University of Munich, Germany, presented his work on supporting teachers to integrate AI into their classrooms. Franz brings a wealth of relevant experience to his research as an accomplished textbook author and K–12 computer science teacher.
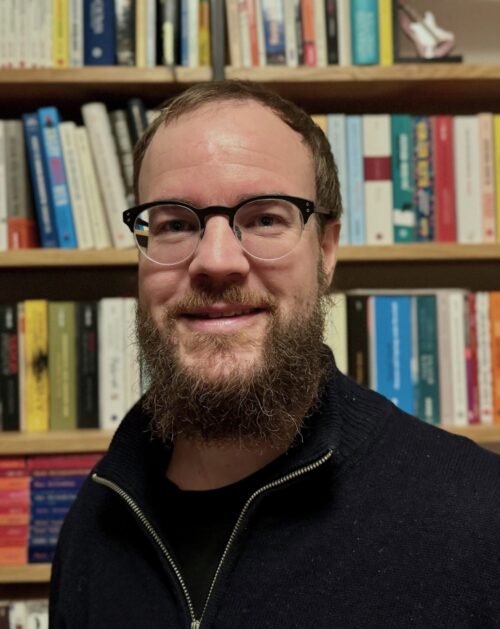
Franz started by demonstrating how widespread AI systems and technologies are becoming. He argued that embedding lessons about AI in the classroom presents three challenges:
- What to teach (defining AI and learning content)
- How to teach (i.e. appropriate pedagogies)
- How to prepare teachers (i.e. effective professional development)
As various models and frameworks for teaching about AI already exist, Franz’s research aims to address the second and third challenges — there is a notable lack of empirical evidence integrating AI in K–12 settings or teacher professional development (PD) to support teachers.
Using professional development to help prepare teachers
In Bavaria, computer science (CS) has been a compulsory high school subject for over 20 years. However, a recent update has brought compulsory CS lessons (including AI) to Year 11 students (15–16 years old). Competencies targeted in the new curriculum include defining AI, explaining the functionality of different machine learning algorithms, and understanding how artificial neurons work.
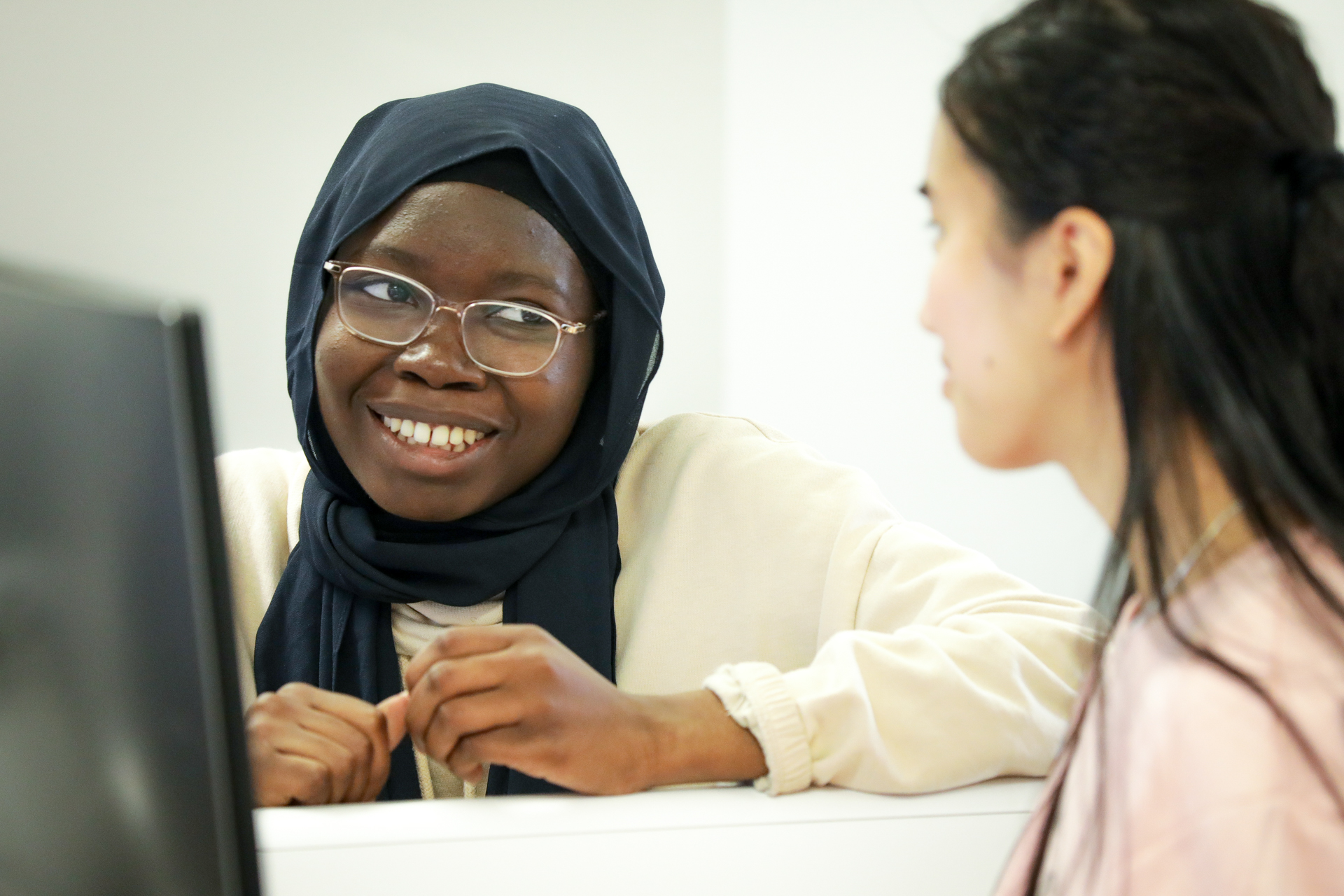
To help prepare teachers to effectively teach this new curriculum and about AI, Franz and colleagues derived a set of core competencies to be used along with existing frameworks (e.g. the Five Big Ideas of AI) and the Bavarian curriculum. The PD programme Franz and colleagues developed was shaped by a set of key design principles:
- Blended learning: A blended format was chosen to address the need for scalability and limited resources and to enable self-directed and active learning
- Dual-level pedagogy (or ‘pedagogical double-decker’): Teachers were taught with the same materials to be used in the classroom to aid familiarity
- Advanced organiser: A broad overview document was created to support teachers learning new topics
- Moodle: An online learning platform was used to enable collaboration and communication via a MOOC (massive open online course)
Analysing the effectiveness of the PD programme
Over 300 teachers attended the MOOC, which had an introductory session beforehand and a follow-up workshop. The programme’s effectiveness was evaluated with a pre/post assessment where teachers completed a survey of 15 closed, multiple-choice questions on their AI competencies and knowledge. Pre/post comparisons showed teachers’ scores improved significantly having taken part in the PD. This is surprising as a large proportion of participants achieved high pre-scores, indicating a highly motivated cohort with notable prior experience teaching about AI.
Additionally, a group of teachers (n=9) were invited to give feedback on which aspects of the PD programme they felt contributed to the success of implementing the curriculum in the classroom. They reported that the PD programme supported content knowledge and pedagogical content knowledge well, but they required additional support to design suitable learning assessments.
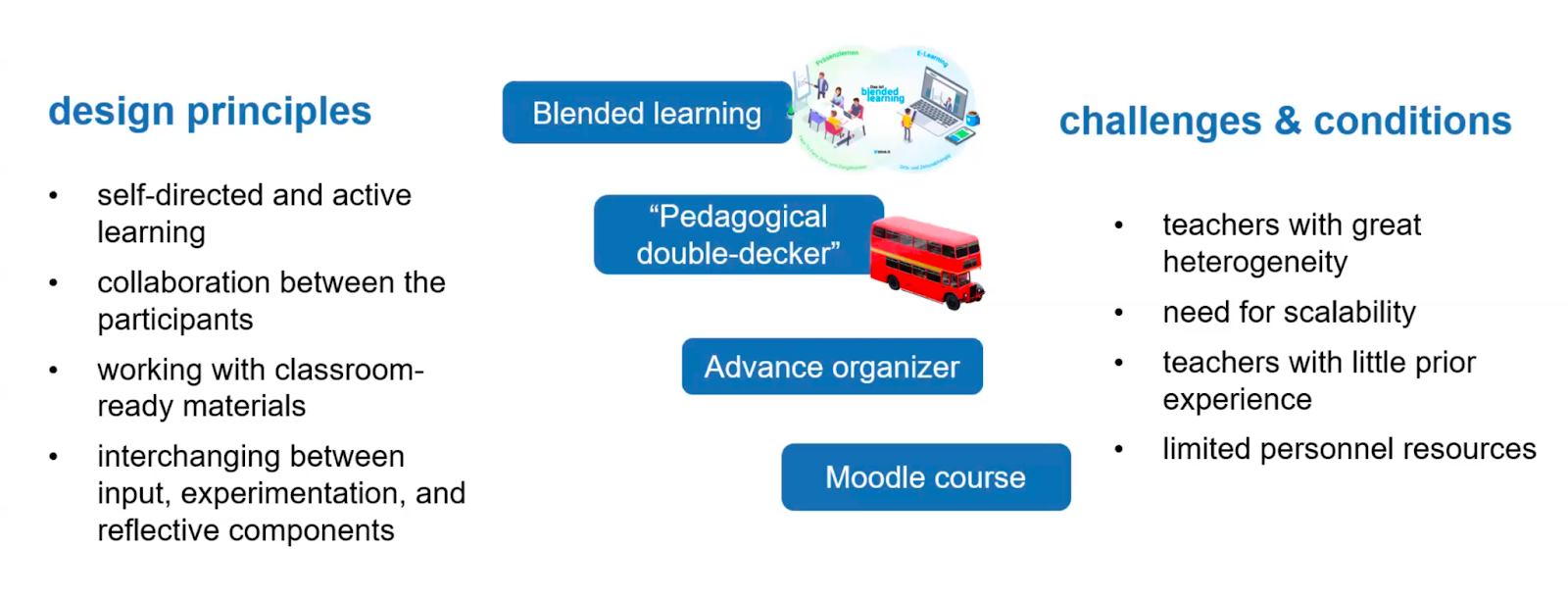
Using action research to aid AI teaching
A separate strand of Franz’s research focuses on the other key challenge of how to effectively teach about AI. Franz engaged teachers (n=14) in action research, a method whereby teachers engage in classroom-based research projects. The project explored what topic-specific difficulties students faced during the lessons and how teachers adapted their teaching to overcome these challenges.
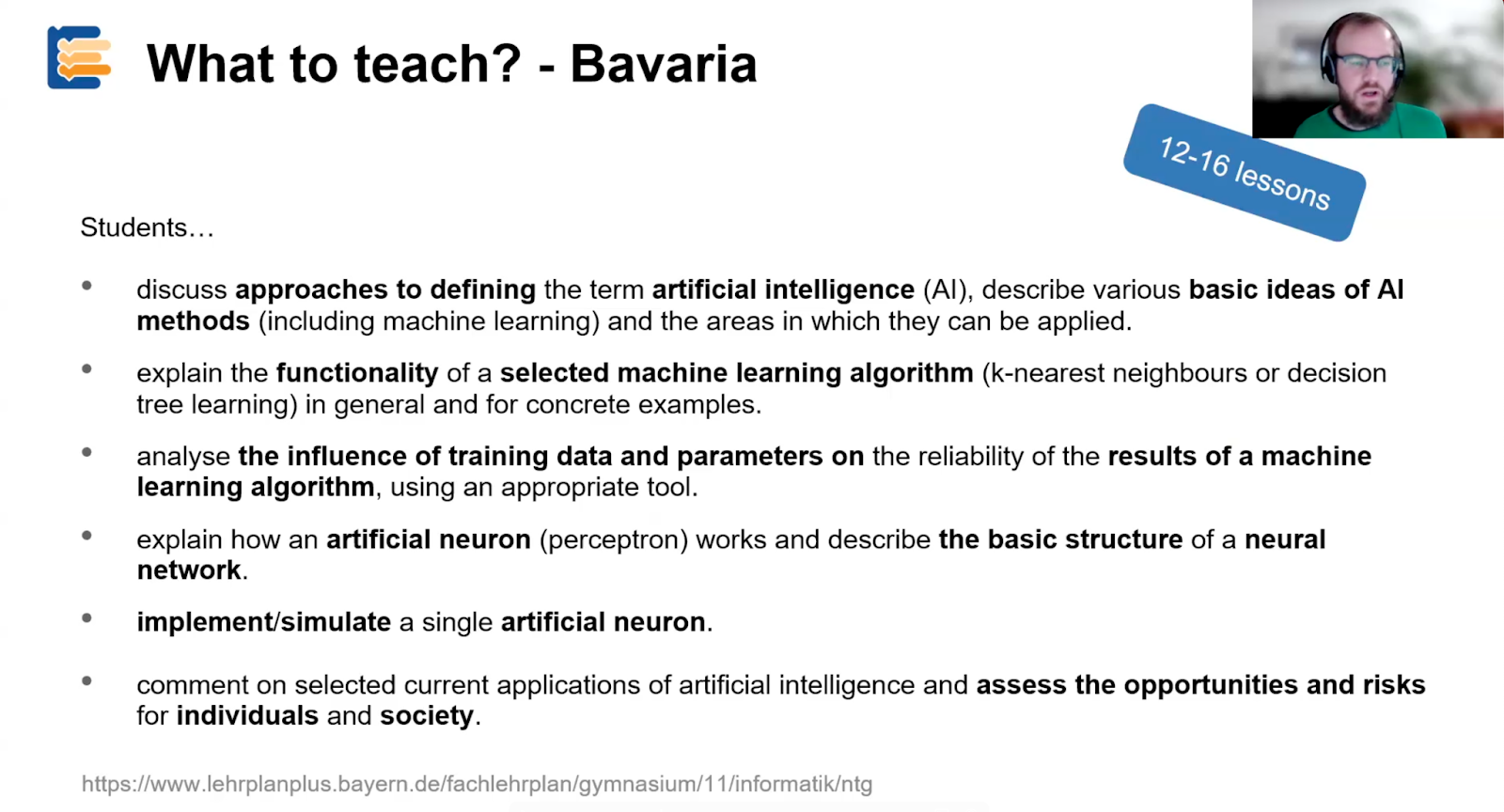
Findings revealed that students struggled with determining whether AI would benefit certain tasks (e.g. object recognition, text-to-speech) or not (e.g. GPS positioning, sorting data). Franz and colleagues reasoned that students were largely not aware of how AI systems deal with uncertainty and overestimated their capabilities. Therefore, an important step in teaching students about AI is defining ‘what an AI problem is’.
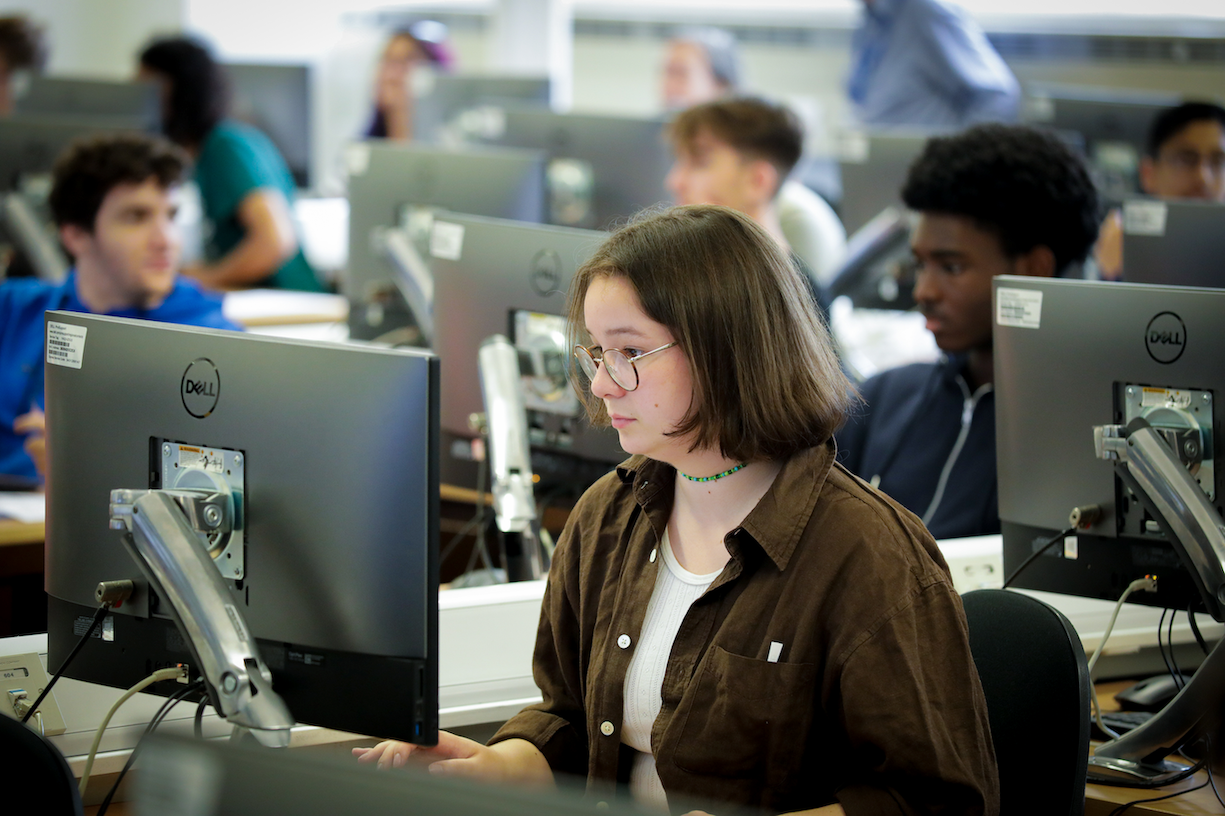
Similarly, students struggled with distinguishing between rule-based and data-driven approaches, believing in some cases that a trained model becomes ‘rule-based’ or that all data models are data-driven. Students also struggled with certain data science concepts, such as hyperparameter, overfitting and underfitting, and information gain. Franz’s team argue that the chosen tool, Orange Data Mining, did not provide an appropriate scaffold for encountering these concepts.
Finally, teachers found challenges in bringing real-world examples into the classroom, including the use of reinforcement learning and neural networks. Franz and colleagues reasoned that focusing on the function of neural networks, as opposed to their structure, would aid student understanding. The use of high-quality (i.e. well-prepared) real-world data sets was also suggested as a strategy for bridging theoretical ideas with practical examples.
Addressing the challenges of teaching AI
Franz’s research provides important insights into the discipline-specific challenges educators face when introducing AI into the classroom. It also underscores the importance of appropriate professional development and age-appropriate and research-informed materials and tools to support students engaging with ideas about AI, data science, and machine learning.
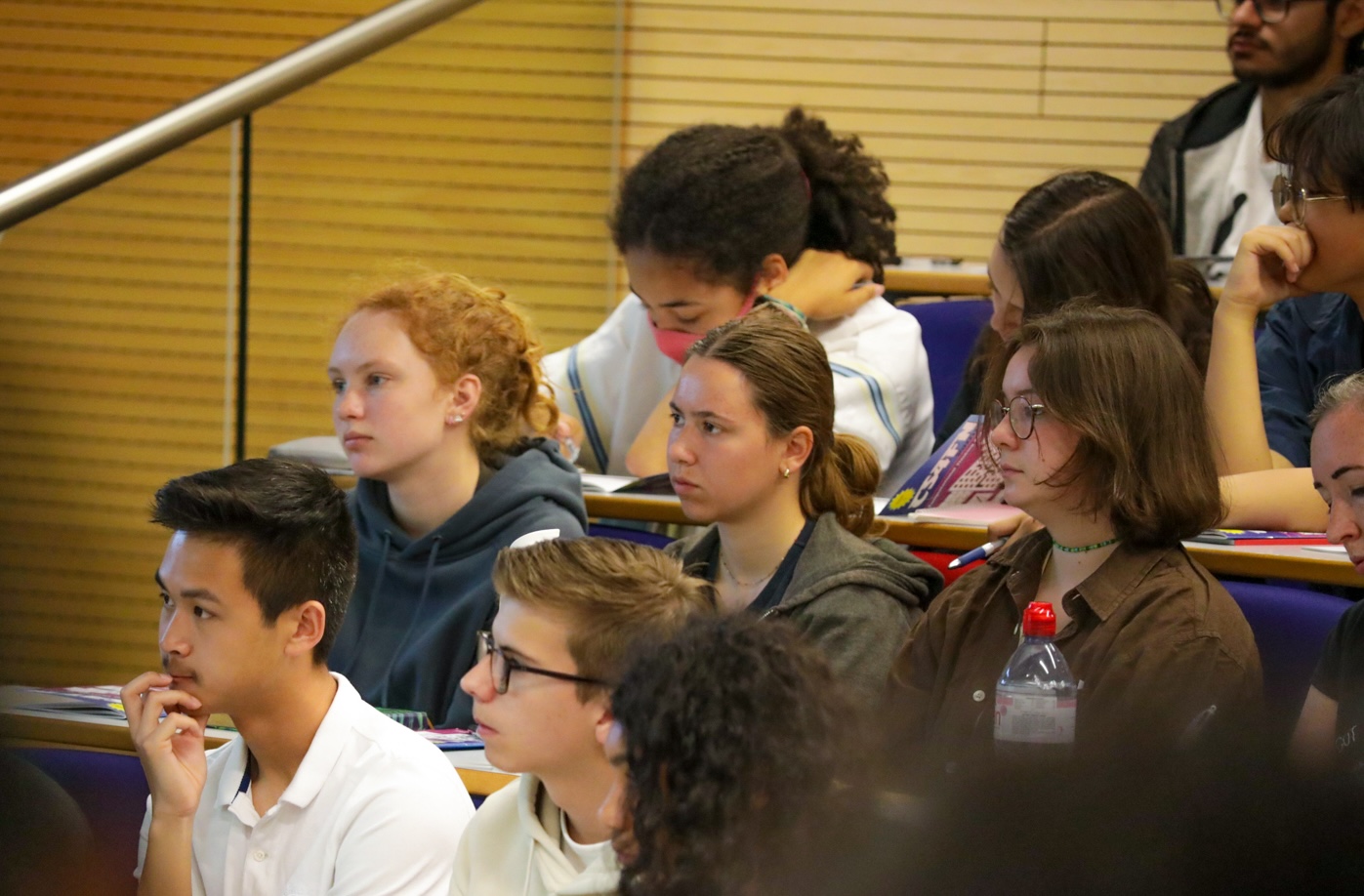
Further reading and resources
If you are interested in reading more about Franz’s work on teacher professional development, you can read his paper on a scalable professional development offer for computer science teachers or you can learn more about his research group here.
Join our next seminar
In our current seminar series, we are exploring teaching about AI and data science. Join us at our next seminar on Tuesday 8 April at 17:00–18:30 BST to hear David Weintrop, Rotem Israel-Fishelson, and Peter F. Moon from the University of Maryland introduce ‘API Can Code’, an interest-driven data science curriculum for high-school students.
To sign up and take part in the seminar, click the button below; we will then send you information about joining. We hope to see you there.
The schedule of our upcoming seminars is online. You can catch up on past seminars on our previous seminars and recordings page.
No comments
Jump to the comment form